Decoding AI: A Chat with Veteran Dr. Nhamo Mtetwa
22 Dec 2024
Reading time: 3 minutes
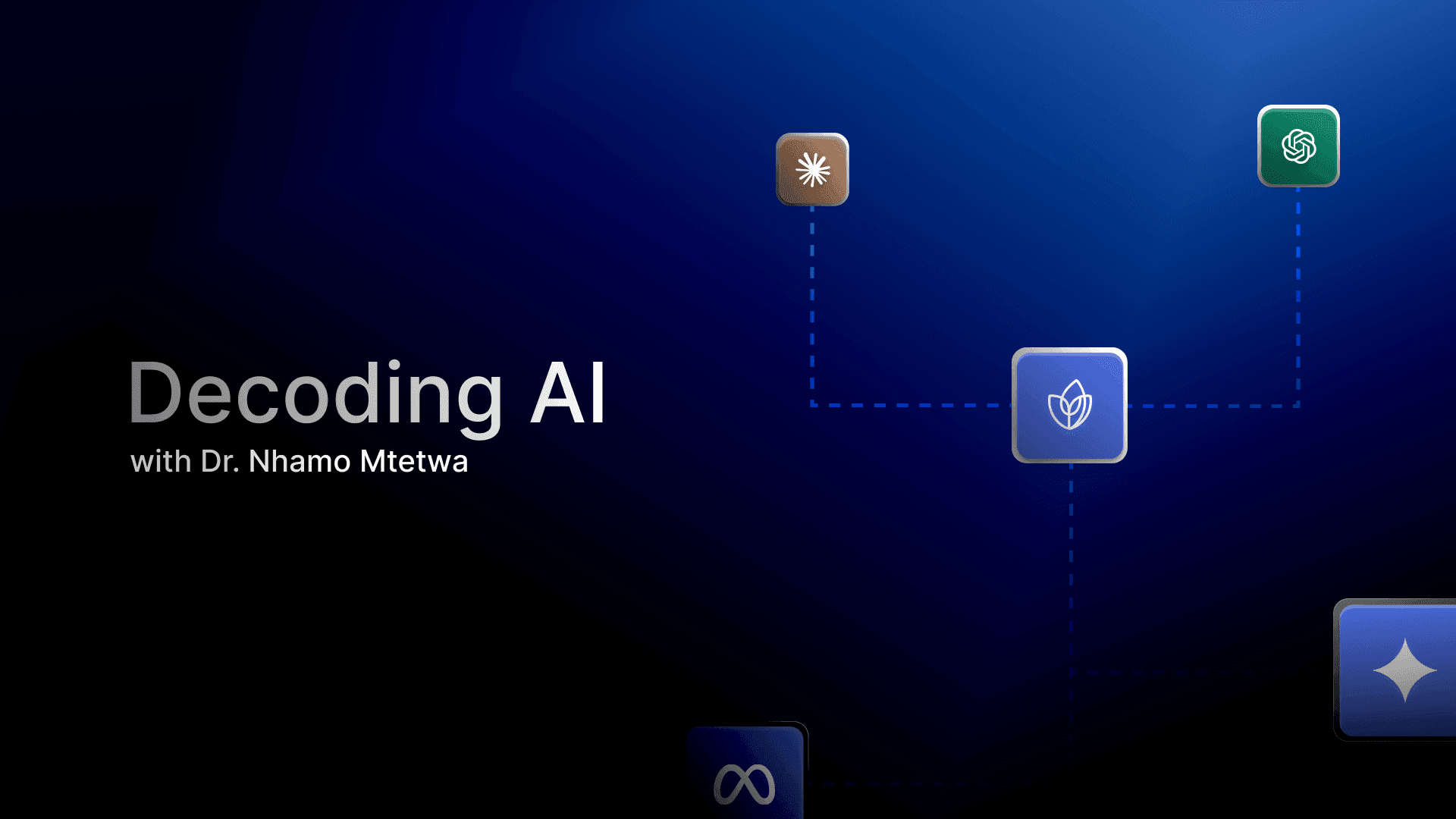
In the rapidly evolving field of artificial intelligence (AI), few individuals have witnessed its transformation as closely as Dr. Nhamo Mtetwa. Since 1999, his journey has spanned both academia and industry, including notable roles at JP Morgan and Barclays. In a recent conversation, Dr. Mtetwa shared profound insights into leveraging Large Language Models (LLMs), the challenges of fine-tuning, and the imperative of continuous learning.
1. Leveraging Large Language Models (LLMs) for Data Enrichment
Dr. Mtetwa highlighted the efficiency of LLMs in streamlining traditionally labor-intensive tasks like data curation. While many focus on deploying machine learning models, the foundational need for high-quality data often takes precedence.
💡 Key Insight: LLMs have reached a point where they can significantly aid in the data enrichment process, helping organizations prepare robust datasets for machine learning applications.
2. Strategic Approaches to Model Fine-Tuning
A common misconception in AI is the necessity of fine-tuning models for every use case. Dr. Mtetwa offered a more strategic approach:
Start with RAG:
Retrieval-Augmented Generation (RAG) systems allow you to inject proprietary data into a model before considering fine-tuning.
Assess Results:
Evaluate the performance of the RAG-enhanced model. Fine-tuning should only be pursued if the additional effort and resources will yield significant efficiency gains.
💡 Key Insight: Fine-tuning is time-consuming and resource-intensive. Start with RAG systems to test viability before committing to fine-tuning.
3. Choosing the Right AI Model: Open Source vs. Proprietary
When it comes to selecting an AI model, Dr. Mtetwa emphasized the importance of aligning choices with specific use cases:
Open-Source Models:
Ideal for regulated industries or when cost control is critical. Self-hosting provides greater flexibility and customization.
Proprietary Models:
Leading providers like OpenAI, Anthropic, and Google offer models with superior throughput and performance, which may be better suited for general use cases.
💡 Key Insight: In scenarios requiring finite accuracy, like healthcare or finance, proceed cautiously, even with advanced models. Tools like RAG are reducing reliability issues but haven’t eliminated them entirely.
4. The Imperative of Continuous Learning in AI
One of the most inspiring aspects of our conversation with Dr. Mtetwa was his ongoing commitment to learning. Despite decades of experience, he emphasized the importance of:
Staying updated on emerging technologies.
Actively engaging with new tools and methodologies.
Embracing a hands-on approach to mastering new developments.
💡 Key Insight: Continuous learning is essential for navigating AI's rapid evolution and unlocking its full potential.
Closing Thoughts
Our conversation with Dr. Mtetwa was a masterclass in understanding AI’s practical applications and strategic use. From leveraging LLMs for data enrichment to navigating the complexities of model selection and fine-tuning, his insights serve as a valuable guide for anyone in the AI space.
As we at Petals continue to explore the possibilities of AI, we are reminded of the importance of adaptability, learning, and thoughtful implementation. By staying informed and open to emerging technologies, we can harness AI’s potential to drive innovation and efficiency across industries.